Key takeaways
- Electricity is one of the most powerful inventions in human history. It transformed every aspect of society, from communication and transportation to industry and entertainment. It also enabled the creation of new technologies that revolutionised the world of finance, such as telegraphs, stock tickers, calculators, and computers
- Today, we are witnessing a similar transformation with the advent of artificial intelligence (AI), especially generative AI, which can create new features that were previously unimaginable
- Generative AI is making an impact at Man AHL, from data augmentation and feature engineering to data extraction and portfolio construction
Introduction
Today, it is difficult to imagine a world without power at the flick of a switch. Yet 20 years after the electric light bulb was invented by Thomas Edison in 1879, just 3% of US households had electricity. It took another two decades for mass adoption. This point is well made by Agrawal and his colleagues in ‘Power and Prediction’1 where they argue we are at a similar juncture in AI. We find ourselves in ‘The Between Times’, where there is plenty of enthusiasm while we await a truly game-changing application.
At Man AHL, we observe somewhat similar trends. Generative AI has certainly not yet replaced researchers or portfolio managers, or generated a whole new system for delivering market beating performance. What it has done, however, is boost productivity, allowing quantitative analysts (‘quants’) to spend more time focused on alpha generation. In this article, we showcase four examples of generative AI making an impact. We also discuss the challenges and opportunities of generative AI for the future of quant research.
Why the sudden uptick in AI hype?
The current focus is predominantly on generative AI. This leap allowing users to interact with models using human language and generate new outputs has been a significant driver of the recent excitement. Generative AI is a subset of machine learning, which is a subset of broader AI. (Figure 1).
Figure 1. Subsets of Artificial Intelligence
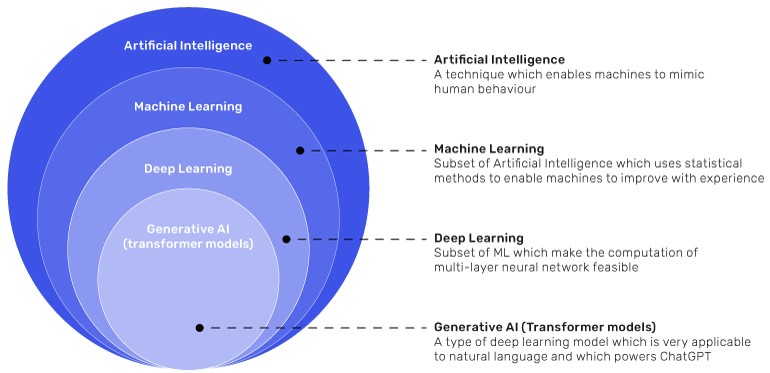
Schematic illustration. Source: https://www.researchgate.net/figure/A-comparative-view-of-AI-machine-learning-deep-learning-and-generative-AI-source_fig1_373797588
For interested readers, the evolution of generative AI is covered in detail here2 with a discussion on the history, how it works and forecasted economic impact. Machine learning techniques are already also well documented in asset management, with success enhancing asset predictors, improving risk management and driving down costs of execution.3 Here at Man AHL, we have been using machine learning techniques3 for over a decade and are early adopters of generative AI.
Four ways we are using generative AI today:
We have a feeling generative AI will scale more quickly than the lightbulb of 1879. Our CIO recently detailed the adoption rate at Man Group4 (spoiler, it’s more than 70% of employees) while our Group CEO also discussed the efficiency gains here5. Below we show four ways generative AI is making us more productive.
#1: Coding with Copilot – interacting with code using natural language
One of the most effective use-cases of generative AI is assisting with coding. Tools like GitHub Copilot can accelerate the development of working prototypes and initial research results by predicting code continuations, reducing development time. It also facilitates knowledge sharing, as developers can ask the AI to explain various parts of code written by others.
The challenge and the opportunity lie in training these tools to understand proprietary internal code. At Man AHL, we have extensive libraries of proprietary code for tasks like market data acquisition, ticker mapping, and running simulations. Off-the-shelf AI models lack knowledge of these specialised repositories.
We are developing chatbots with the capability to comprehend our internal code. For example, one chatbot can identify where to find metadata for a market code and retrieve timeseries prices, specifying the correct libraries and fields, saving time. It is a significant challenge which requires a lot of work to get useful outputs, but this capability enhances our efficiency and leverages our proprietary knowledge (Figure 2).
Figure 2. Copilot plotting a timeseries of S&P 500 E-Mini Future, using our internal libraries
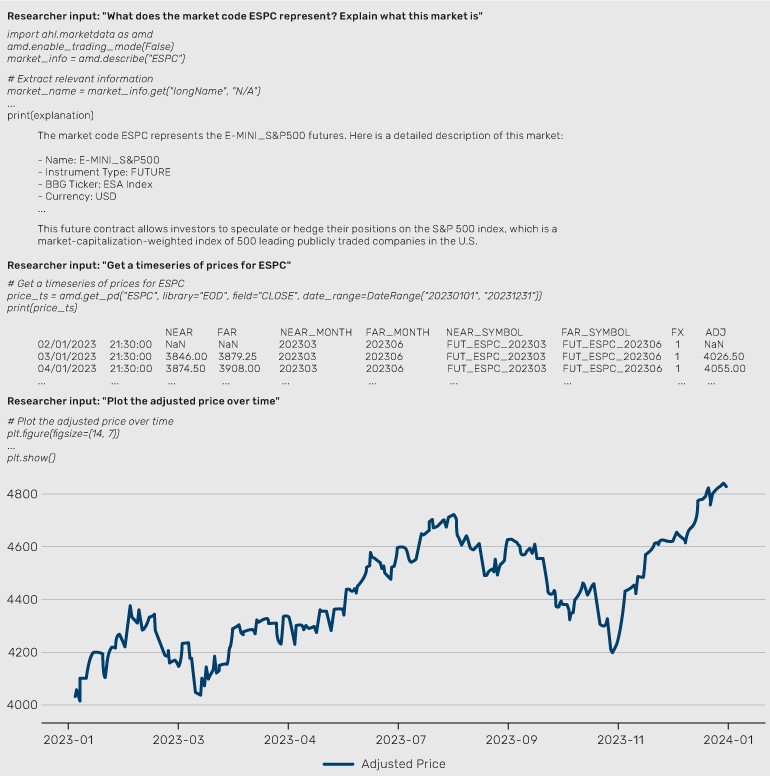
Schematic illustration.
#2: Extracting information for trading catastrophe bonds
Man AHL was founded as a commodity trading advisor (CTA) trading futures contracts. Futures are highly standardised and liquid, making them easy to trade for a systematic investment manager. However, as we’ve grown and diversified our business, we increasingly trade more novel and exotic instruments.6
One example is catastrophe bonds, which are debt instruments designed to pay out when a pre-specified event occurs, typically a natural disaster. Each catastrophe bond has unique features which need to be clearly understood before investment and, unlike interest rate or credit default swaps, do not have standardised terms. This process involves reading the offering circular, which is done by a human analyst, and a second check of the extracted data, again by another human analyst. As these documents run to 200 pages, this can be a considerable amount of time (Figure 3).
Figure 3. The offering circular for a catastrophe bond can run to more than 200 pages
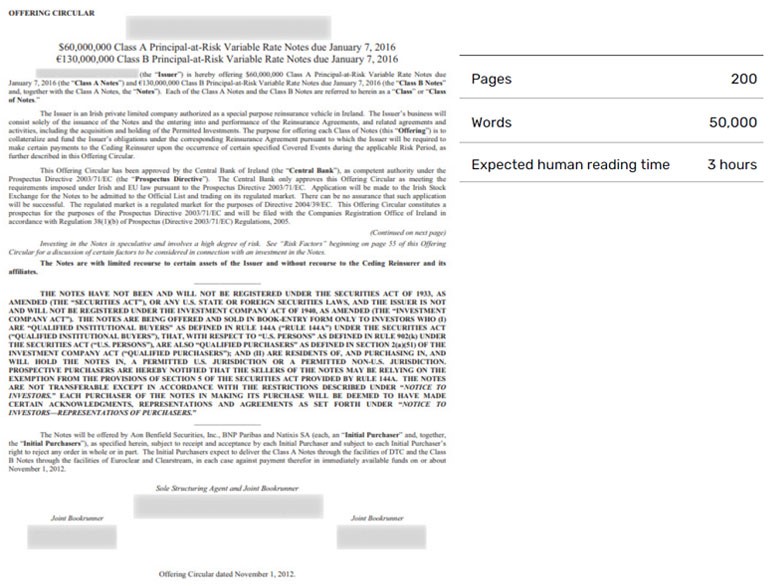
Schematic illustration.
Today, we are testing a process where this data extraction is done by ChatGPT, putting the relevant information in a systematic template for a reviewer to check. This frees up one analyst to focus on new research.
#3: Chatbots assisting with research for investor queries
Man Group’s Client Relations team assists with questions from clients on the firm’s full suite of investments, including Man AHL’s systematic investment strategies. Many questions rely on information from various investment materials, including factsheets, presentations, due diligence questionnaires, and investment commentaries. The team then crafts client-friendly responses. For example, a client might request information on fees, the number of markets traded, or the risk target of an investment strategy
ChatGPT can automate several steps in this process. First, it can extract the required information from the relevant documents. Second, it can draft a response ready for human analyst review. This efficiency frees up time for the team to focus on higher-value tasks. Figure 4 shows a screenshot of the Q&A chatbot querying a document and generating a response ready for checking.
Figure 4. Screenshot of chatbot returning information
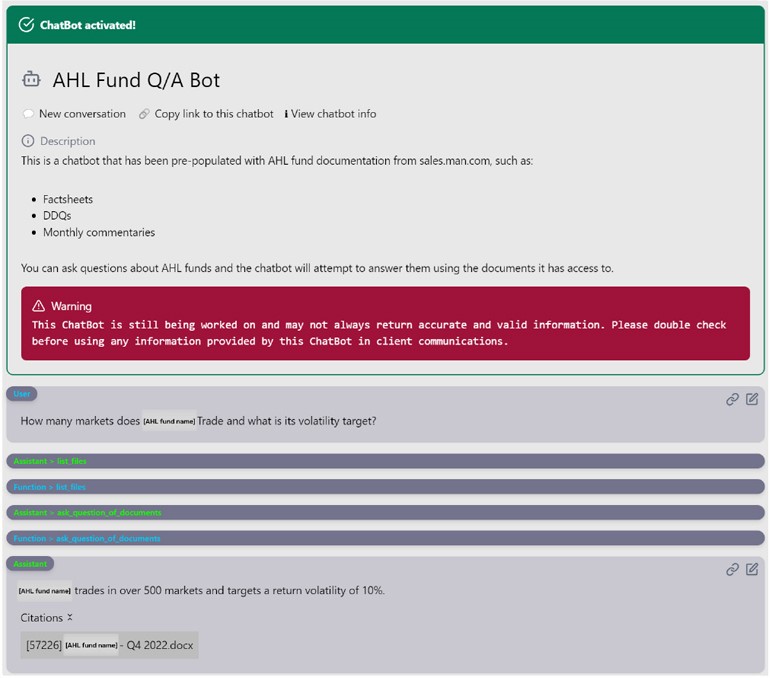
Schematic illustration.
Improved efficiencies for data extraction are a general theme – we saw this in the catastrophe bond example – and other teams are reaping benefits too:
- Discretionary investment analysts extracting information from Regulatory News Service (RNS) announcements.
- Data science professionals extracting data update information from vendor emails.
- Design professionals extracting underlying data from unformatted charts to convert to Man Group branding.
#4: Analysing macro data at the level of a junior quant
ChatGPT is useful in quantitative macro research by leveraging its knowledge of fundamental macroeconomic relationships. One use-case is employing it as a hypothesis generator to suggest whether a particular economic timeseries has a fundamentally justifiable relationship with a certain market. These hypotheses can then be tested using statistical back-testing methods.
While ChatGPT won’t replace our macro research team in its current state, its understanding can be as good as a graduate researcher. The main difference being that a human researcher needs breaks, while ChatGPT can query thousands of relationships systematically, and potentially suggest signals on those relationships.
ChatGPT also speeds up learning fundamental macro information. Compared to hand-crafted resources like Wikipedia, ChatGPT can be more concise and relevant, helping researchers quickly understand key drivers of macroeconomic phenomena.
Figure 5. Illustration of ChatGPT explaining a simple macroeconomic relationship
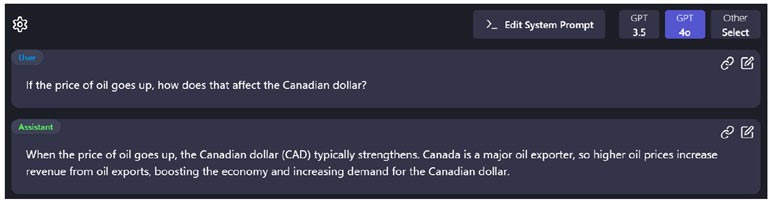
Schematic illustration.
What have we learnt so far?
We’ve focused on the opportunities until now. Below we highlight some of the key lessons from our experience with generative AI.
Hallucinations must be managed
ChatGPT’s responses cannot be fully trusted. To help mitigate the impact of hallucinations, we use tools to highlight where information occurs in the original text, aiding human checking. It is a similar story for code, which is only a prototype and requires human verification.
Prompt engineering is crucial
If ChatGPT can’t do a task well, it’s often due to a mis-specified prompt. Perfecting prompts requires significant resources, trial and error, and specific techniques.
Break tasks into smaller sub-tasks
ChatGPT can’t logically break down and execute complex problems in one go. Effective ‘AI engineering’ involves splitting projects into smaller tasks, each handled by specialist instances of ChatGPT with tailored prompts and tools. The challenge is integrating these agents to solve complex problems.
Education is key for wider adoption
Understanding ChatGPT’s capabilities and limitations is crucial. Sceptics should see its strengths, while enthusiasts need to learn its failures. Effective use requires learning how to interact with the model and understanding its training and functioning.
Be ready for the next best thing
Generative AI is already creating efficiencies in asset management, but users must be agile in taking on the next best model to reap the gains of this evolving technology. Progress has so far been swift, with GPT-2 released in 2019 described as ‘far from useable’7 versus GPT-4 which is already gathering multiple use-cases and appears to be scaling faster than historical technologies.
Conclusion
Electricity changed society but took 40 years to do it. AI can do the same,8 but faster. Our analysts have already seen improvements in data augmentation, feature engineering, model selection and portfolio construction. We believe that in as little as a decade, those asset managers who embrace generative AI can help gain a competitive edge via a faster pace of innovation and superior performance.
Our investment writers are also upskilling in using generative AI tools. As Luis von Ahn, CEO of Duolingo, noted: “your job’s not going to be replaced by AI. It’s going to be replaced by somebody who knows how to use AI.”9 Check out the ’Key takeaways’ again and we can see firsthand the talents of GPT-4 and how our writers are taking this advice to heart.10
It was 40 years from Edison’s lightbulb until electricity changed the game for the masses. Perhaps AI hits that milestone in ten.
Harry Moore is a Principal, and Martin Luk is a Quant Researcher at Man AHL. Matthew Hertz is Head of Machine Learning Technology at Man Group. Man is a fund manager partner of GSFM, a Firstlinks sponsor. The information included in this article is provided for informational purposes only.
For more articles and papers from GSFM and partners, click here.
References
1 Ajay Agrawal, A., Gans, J. and Goldfarb, A. ‘Power and Prediction: The Disruptive Economics of Artificial Intelligence’ (2022).
2 Luk, M., ‘Generative AI: Overview, Economic Impact, and Applications in Asset Management’, 18 September, 2023.
3 Ledford, A. ‘An Introduction to Machine Learning’, 2019.
4 Korgaonkar, R., ‘Diary of a Quant: AI’, 2024.
5 Pensions and Investments, ‘Man Group CEO sees generative AI boosting efficiency, but not investment decisions’, 30 April 2024.
6 Korgaonkar, R., ‘Diary of a Quant: Journeying into Exotic Markets’, 2024.
7 Radford, A., Wu, J., Child, R., Luan, D., Amodei, D. and Sutskever, I., ‘Language models are unsupervised multitask learners’, 2019. OpenAI blog, 1(8), p.9.
8 Eloundou, T., Manning, S., Mishkin, P. and Rock, D., 2023. ‘Gpts are gpts: An early look at the labor market impact potential of large language models’. arXiv preprint arXiv:2303.10130.
9 Bloomberg, Odd Lots podcast, ‘How Humans and Computers learn from each other’, 2 May 2024.
10 Key takeaways generated by ChatGPT, using Copilot for Word.